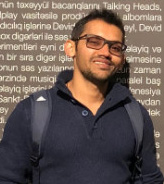
We live in a world in which data is growing exponentially, and more and more effort is going into making better use of it. Marketers today have more than enough data available to them, but they’re looking for better ways to use and connect their data sets to gain deeper, more valuable insights.
The challenge lies in the way we look at the application of data science and the way we as data scientists have been trained. Working over the years on data science and analytics projects, I have been able to see an easier way to achieve bigger, more audacious goals.
It’s a four-step process:
- Start with the ambitious outcome
- Break the outcome into smaller action sets
- Explore the data and run the analysis (iterative)
- Tie the findings to the set outcome
This process works better than the traditional data science problem-solving process because it reduces ambiguity and fuels drive for outcome with everyone involved. The traditional data science process typically starts with identifying a problem statement, then moves toward data collection, data modeling and evaluation. At the heart of traditional data science process is complexity. Complexity generally forces us to think more rationally, analytically and does not make us use our creativity. Ambition on the other hand encourages us to start with creative thinking and then move towards our analytical side of the brain.
Now let’s break our process into the individual steps.
Start with the ambitious outcome
Let’s say you want to achieve 5% additional share in the next year. First, define your ambitious outcome. In this case, 5% more share. Behavioral psychology says it’s important to reframe the goal positively because it tricks your mind to think more creatively in order to achieve the goals.
In this example, let’s say you want to increase share by expanding your distribution network in Indonesia. Indonesia is a very fragmented country geographically, with 17,000 islands, and transportation is a challenge. In order to achieve this, the objective is to reach from 50% to 80% of the market at the lowest possible cost and all this in the span of a year.
Break your outcome into smaller action sets
This is critical because you want to break your big ambitious outcome into smaller manageable sets. It also helps to put your data into “mutually exclusive collectively exhaustive” sets. This helps to divide it into multiple sub-objectives and fast-track the process. In our example, we divided the outcome into three action sets:
- Dense geographies requiring distributor setup
- Scattered geographies requiring sub-distributor setups
- Growing population clusters
Explore data sets and run the analysis
This is a big part of the process. Start by exploring data sources and listing what is available and what is not (but is a must-have). In this example, we can use Nielsen retail census and retail panel data with different features like store size, store sales, store location coordinates, etc. We can then integrate these two data sets to arrive at a number of meaningful features.
However, we also recognize where our data sets fall short. In this case, we might find that there is a sizeable area outside our retail scope. As a result, we might not have certain types of information, like population, number of banks, which can indicate prosperity, and other data. So we must identify other sources such as population censuses to get these features.
These data sets are then fused and run through a multiple regression model, which identifies the opportunity for each area/locality.
A few iterations are likely required to ensure the accuracy of the prediction. Finally, this data can be merged with client or first-party distribution data.
Tie the findings to the outcome
The final step is to tie all the outcomes of the action sets together.
In this example, we are then able to use this data to draw area/block clusters that deliver the most opportunity and can be realistically supported by the existing distribution network with only a slight increase in their radius and setting up of few sub-distributors in growing clusters.
In this example, we’re able to combine multiple data sets to deliver a simple solution that outlines a clear solution to a large, ambitious goal. Which goes to show that it doesn’t always pay to start off with a problem statement, but rather a positive ambitious goal.
So next time you start with a new project, give this process a chance and start with an ambitious goal and creative thinking.